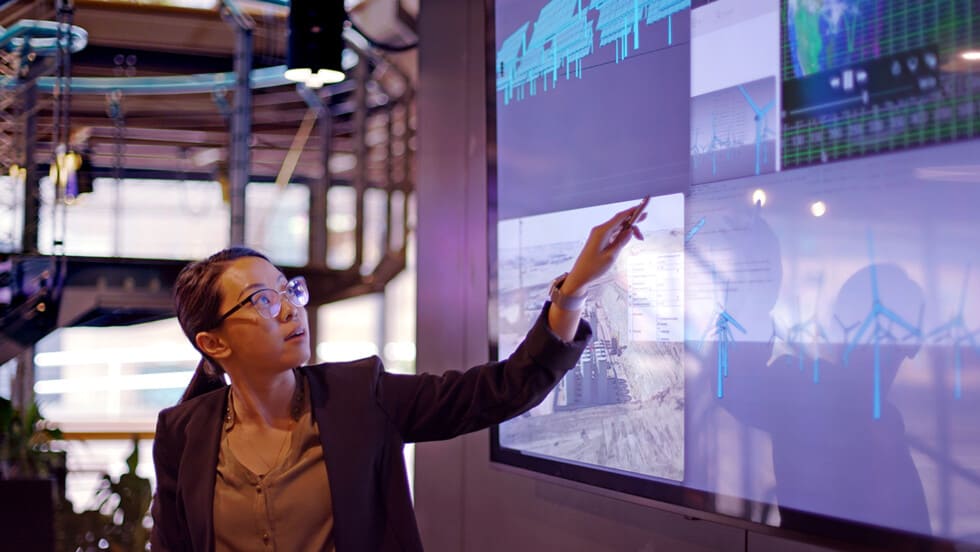
Execution, however, is where plenty of challenges persist. Foundry’s 2022 Data & Analytics study identified a broad set of barriers to success, including a shortage of appropriate skill sets, poorly articulated data strategy, and a pair of related funding issues: lack of budget and difficulty demonstrating ROI for data initiatives. Tactical pain points include data quality, security and governance, the ability to analyze data, and data integration.
Overcoming these issues starts with a recognition that your data strategy is a continuous journey, given the persistent and significant growth in data, rapidly evolving technology, and shifting market and customer needs.
“The data strategy needs to be evergreen, because technology and architecture — which are pushing the boundaries of what’s possible — do change,” says Arthur Hu, Senior Vice President, Global CIO, and CTO of the Solutions and Services Group at Lenovo. Successful execution of data strategy, Hu advises, depends on four key areas: the data foundation, data governance, artificial intelligence (AI), and metrics.
Start with a solid data foundation
Lenovo’s own data strategy began to take shape more than a decade ago, when the company decided to move from a federated IT model to a more integrated approach with centralization around key strategic elements, including:
- A solid data platform
- A coherent data strategy
- A standardized data taxonomy
- Common data models
The data platform is the first essential element,” Hu says. “It’s the clearinghouse or hub that will give access to those who will be using the data.”
Common data objects and data definitions are important because they give all stakeholders “a shared currency and language without having to reinvent the wheel,” Hu says. Shareable, standardized data models enable people to put data to use more quickly because they don’t need to clean or validate it every time they want to use it.
But that doesn’t mean the data remains static. “It’s important to keep in mind that you never have a perfect data architecture,” Hu says. “You should constantly be thinking about what to bring in and what to retire.”
Keep data governance in your sights
Data governance is another constantly evolving component. “Governance is pivotal to enabling a data strategy, but it’s really hard work,” Hu says. “It’s not just about technical governance, but business governance as well. It’s about making sure the people who generate the data are held accountable and have the right KPIs. It’s not the most glamorous thing, but it’s the piece that holds everything together.”
For business stakeholders, data governance comes down to identifying the value data is generating and putting proper controls in place to protect the company and ensure compliance. As organizations become more global, they must pay particular attention to data sovereignty issues to keep up with ever-changing regulations about personal identifiable information and other sensitive data.
“One critical area is how information is stored, transmitted, and processed,” Hu says. “Keep in mind the desired future architecture so you’re able to maximize the benefits of getting data together. At the same time, know that not all the data can go to all the places you’d like at the same time.”
Demystify artificial intelligence
Modern data strategies have been buzzing around the use of AI and machine learning technologies for processing, analyzing, and delivering insights from data. It’s important, however, for CIOs to separate the mystique of AI from the realities of deploying it.
“AI is not magic dust,” Hu says. “There is no such thing as ‘I put AI on the data and great things happen.’ AI is technology in service of a goal. But when applied properly, it can deliver incredible insights.”
AI can become a key part of the analytics tool kit, as devices across an organization proliferate and generate increasing volumes of data. “There are not enough people or time to look at patterns and discern meaningful ones from all of that data,” Hu says. “In this regard, AI can enable you identify the right information to enable your goals.”
But AI is not sufficient on its own. Hu stresses the need for augmented intelligence, rather than artificial intelligence, with people working with AI outputs to provide important context and guidance for the insights the algorithms are generating. “Augmentation brings together artificial and human intelligence to help organizations gain deeper value,” says Hu. “It’s important to know why you’re getting a particular insight. People need to contextualize it. If you get an insight but it’s not trusted, it’s not going to be used, so you’ve missed the point.”
Measure against business outcomes
Because data strategy is an ongoing journey, it’s critical for CIOs to measure their progress along the way. Hu suggests a combination of quantitative and qualitative metrics that ultimately tie back to business objectives.
“First, you need baseline metrics,” he says. “As a technology team, you want to understand how your data platform delivers on basics like uptime, data accessibility, and user experience, because if those aren’t right then almost certainly, people are unhappy.”
Equally important is measuring business value. Hu recommends keeping a scorecard, using easy-to-understand visuals to help leaders remain focused on the functional, regional, or global objectives that are most important to them.
The first two sets of metrics will contribute to a third qualitative measurement: The perception among senior leadership about whether investments in data platforms and tools are paying off.
“You’ll know you’ve hit the right balance when people’s faces light up and they tell you how regional data helped them develop a new product, or this particular insight increased customer satisfaction scores,” Hu says. “That’s when you’ll know your data strategy is working.”
Data: Want more? Watch the Does your raw data stink? episode of Lenovo Late Night I.T. Season 2.